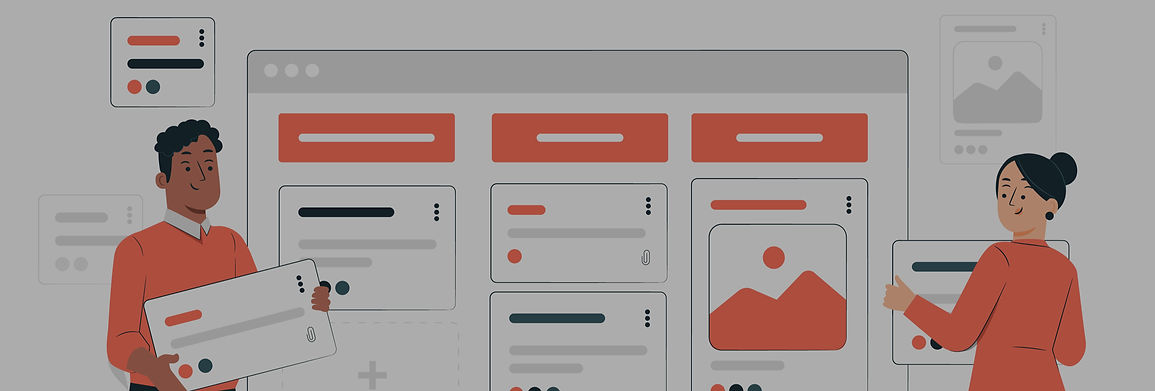
Designed by Freepik
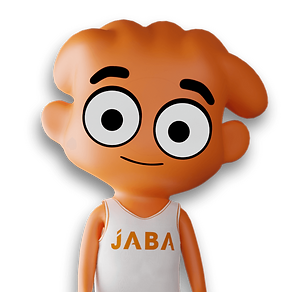
Beta Design
Jaster Athletics
The first stages of development of a generative AI feature for Jaster Athletic's JABA AI mobile app.
Overview
Role
UX Researcher and Designer
Timeline
May 2024 - Present
Deliverables
Journey Maps
Competitor Analysis
UI Mockups
Tools
Canva
Notion
The Problem
JABA’s Caption Generator is an AI feature that was prototyped in Figma, but never developed and put into the app. It was not initially researched through a user experience lens, and clear problems were pointed out by team members. Product management wanted a new approach to this feature; one informed through research to create a feature that is not just another wave in the AI surge, but that can actually be a tool that services the needs of its users.
My Role
I was working as an intern at the time, but when this project came up I was picked by upper management to lead the way. It was a revisitation of a once-abandoned project, which I was able to approach with a fresh set of eyes. I’m thankful for the trust that my superiors had in my perspective, and the opportunity that this project gave me to explore UX research in a startup environment.
I had been an intern at the company for a few months at this point, and was well-acquainted with the existing product and the company’s vision for the future of the product. I was also given the help of a new product design intern to assist with the project. With this new partner, we were able to share in each other’s expertise to gain deeper insights from our research.
Project Goals
-
Share in one another’s expertise to find the best solutions for our users.
-
Gain a complete understanding of the existing screens that had been designed by another team.
-
Design informed by thorough research of our competitors and user needs.
-
Plan for recursive research by exploring a wide variety of options up front.
-
Design that considers the product audience of college athletes.
-
A final product that is intuitive and easy to use for any AI experience level.
-
Document all work along the way in Notion for future reference.
Abstract
Approaching this project, I created a design process for me and my partner to follow, knowing we would tweak it as needed down the line. To get us started, I looked through the company’s Figma files and found the existing screens that had been left abandoned. We created journey maps based on the flows in these screens, so that we could gain an understanding of the current user flow and its pain points. These showed us a significant amount of negative experiences surrounding the current design, pointing out clear issues with these flows.
I think it is important as a startup to reference what other companies were doing, so we checked out some of the competitors in the caption generator space. We proceeded to conduct a competitor analysis, noting what criteria each competitor had available. We wanted our final product to offer everything that most competitors did, as to properly compete with them, and go beyond those criteria to take our own spin on the feature.
The existing product had some aspects to it that were unique to our users (namely, college-age, returning users, with their socials linked in the app) that we found to be innovative and set us apart from our competitors. These steps informed our acceptance criteria, a list of all aspects that we wanted to include in the feature. The question then became, how do we gather and present all of this data in the most simple, time-efficient way? We conducted wireframing, and based on that I made some mockups to show what our ideas would look like on a more concrete level. This is where the project stands today, as we prepare for A/B testing with our mockups. I see user research as vital in this stage of the process, and don’t want to move forward without some data to point us in the proper direction.
Let's get into the details
Existing Materials
We were given a prototype of 17 screens, and were not able to get in contact with the designer who had worked on these. There was no other documented work other than this prototype. Myself and my partner spent the time to fully understand the user flow and system design behind these screens.
This existing design is also visually outdated from the current app. The app has since gone through an aesthetic shift, so the final design will need to follow this updated style.

Journey Mapping
In order to further understand the current prototype and the user’s interactions with it, we decided to turn to journey mapping. This let us see how a potential user may experience the flow, and it showcased the pain points of the current user flow.
The existing prototype splits off into four different flows, depending on which generative experience the user selects in the initial screens. We created journey maps for each of these four different flows to compare with each other, and search for any commonalities.
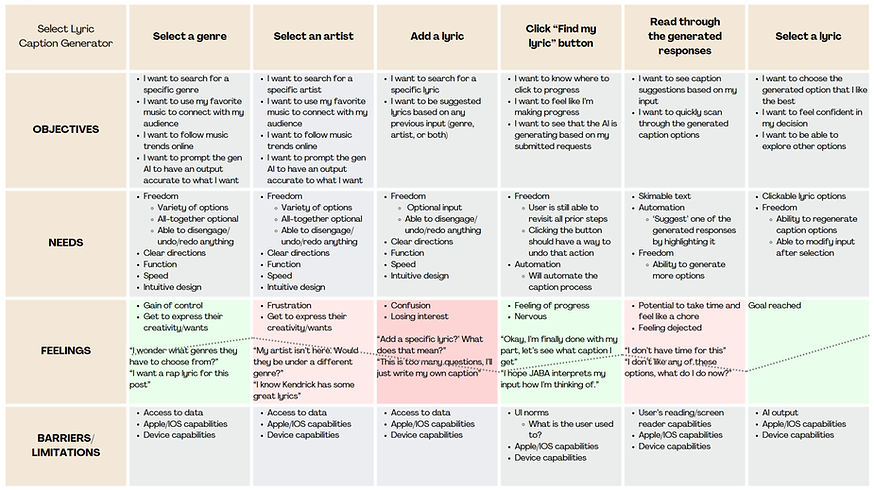_edited.jpg)
1/4 Journey maps created for the lyric generation flow.
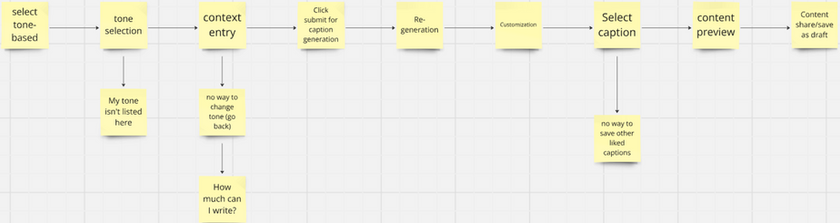
Additionally, user flows are created to map out the current prototype's user experience.
Findings
We found that the experience with the current system has the potential to bring up many negative feelings in our users. At many points they could feel confused, conflicted, frustrated, bored, and like their time is being wasted.
The causes of these negative emotions are generally moments where the user needs to read through a decent amount of text, make too many selections, doesn’t receive feedback from the app (as in a loading icon showing their their requests are being processed), doesn’t understand the prompt, or doesn’t see their desired input as an option on the screen.
Competitor Analysis
With the state of the product, the app does not have any users. This limits the user research methods available to us. With this in mind, competitor analysis is a great way to inform our design process. Assuming that the competitors we look at have conducted proper research to inform their design choices, then common threads between competitors should give us insight into the most effective design choices.
Competitors
Analysis / Comparison
Legend and Notes
.png)
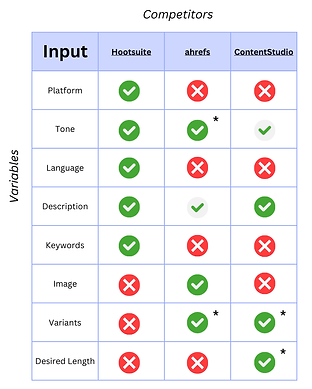.png)
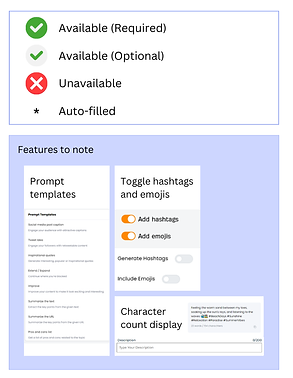.png)
Findings
There is a common setup for user input of the competitors. Both the ahrefs and ContentStudio each have 1 optional field, 3 required fields, and 2 of those required come auto-filled.
Based on the existing prototype, we also noted that our “generate a lyric caption” and “generate based on past caption” options set us apart from our competitors. These generation options consider our audience, with lyric captions being popular among the younger age demographics and past captions being readily available for any user with their social accounts linked in the app.
Overall, there seems to be a balance to strike with generative AI. We want to gather as much information as we can so that we can give the user the most accurate responses. Yet, we also want to keep the process timely and largely-automated, without too much work done by the user. Otherwise, it would defeat the purpose of a generative feature in the first place, being to save the user time and energy.
Acceptance Criteria
To prepare for the designing stage, we first note the acceptance criteria, or product requirements. This will clearly state what the feature must do and show the user. We're not worried about creating an exhaustive list here, but rather are focused on hitting the key points that we want to make sure we hit the final product. These criteria will guide the design process.
Notice that the criteria is coming from both the competitors and existing materials. This is because we want to make sure we are offering the same services as our competitors, and then building on that experience with the criteria that make our version unique.
From competitors
-
Generate based on tone
-
Control over # of variants
-
Generate based on a description
-
Ability to add hashtags or emojis
​
From existing materials
-
Generate based on an image
-
Generate based on past captions
-
Generate lyric caption
​
Overall
-
Clear character caps for all text fields (e.g. 0/200)
-
Clear word and character count of the generated caption (e.g. 23 words / 150 characters)
-
Option to generate based on:
-
Platform (Instagram, Tiktok, etc.)
-
Language
-
Keywords
-
Desired length
-
-
Outputs a caption based on the input
Wireframing
With our acceptance criteria clearly laid out, we moved on to a wireframing phase. We ended up creating wireframes for all four individual flows, as well as one for a proposed combined flow that would house all generation options on one screen.

Wireframing of the flow for generating captions based on tone.
Midfidelity Mockups
At this point, we knew that we needed to move forward with either the branched or condensed version. After wireframing the flows and proposing them each to the larger product team, we determined that we needed to conduct user research on the topic. We knew the safe option would be to resort to the condensed version that our competitors all used. Yet, the condensed option could also overcomplicate the process given that our caption generator has more generation options than our competitors.
We decided to conduct A/B testing with these two versions, and get a real response from our audience of college athletes. In order to have presentable screens for this testing, we next mocked up some mid-fidelity examples of the screens in the wireframes flows. These mockups were all designed by me, to keep the visual design consistent for testing. This is done in an attempt to get specific feedback on the distinct differences between the two versions.
MidFi Mockup
.png)
Test Version: generate caption based on lyric
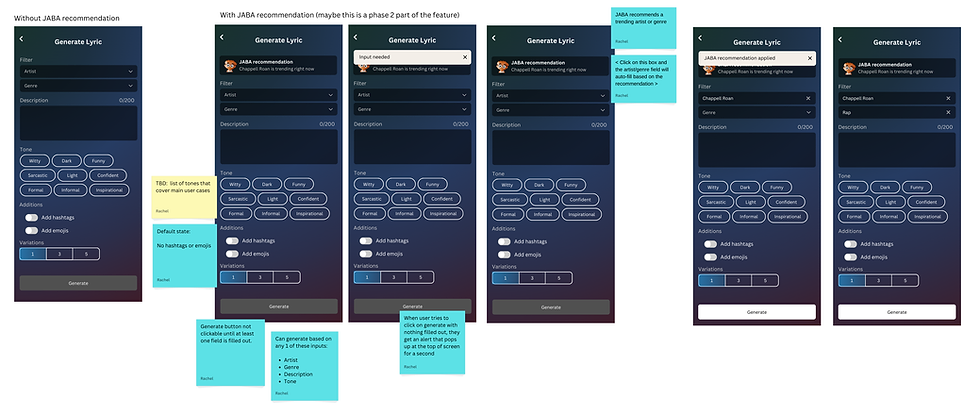.png)
Next Steps
This is an ongoing project, and user testing is still on the horizon. We plan to conduct A/B testing with the mockups, and let that user feedback inform the next round of design.
​
From there, we will go back to the ideation stage, preform more testing and so on. This recursive research is key to well-informed design. The research and design stages can be simultaneous, informing each other along the way.